
LatticeFlow raises $12M to address computer vision blind spots • TechCrunch
LatticeFlow, a startup that was spun out of ETH Zurich in 2020, helps machine learning teams improve their AI vision models by automatically diagnosing problems and improving both the data and the models themselves. Today, the company announced that it has raised a $12 million Series A funding round led by Atlantic Bridge and OpenOcean with participation from FPV Ventures. The round also included existing investors btov Partners and Global Founders Capital, which led the company’s $2.8 million seed round last year.
As LatticeFlow co-founder and CEO Petar Tsankov told me, the company currently has more than 10 customers in both Europe and the US, including a number of large enterprises such as Siemens and organizations such as the Swiss Federal Railways and is currently running pilots with quite a few more. It is this customer demand that has led to LatticeFlow’s growth to date.
“I was in the States and met with some investors in Palo Alto,” Tsankov explained. “They saw the bottleneck we faced with onboarding customers. We literally had machine learning engineers supporting customers, and that’s not how you should run a company. And they said, OK, take $12 million, get these people on board and expand. It was definitely a great moment because when we talked to other investors, we saw that the market had changed.”
As Tsankov and his co-founder, CTO Paval Belik, pointed out, most companies today have a hard time getting their models into production, and then when they do, they often realize they don’t work as well as they expected. The promise of LatticeFlow is that it can automatically diagnose data and models to find potential blind spots. In her work with a large medical company, her tools for analyzing their datasets and models quickly found more than half a dozen critical blind spots in their state-of-the-art production models, for example.
The team noted that it’s not enough to just look at the training data and make sure there’s a diverse set of images—in the case of vision models, which LatticeFlow specializes in—but also learn the patterns.
LatticeFlow’s founding team (from left to right): Prof. Andreas Krause (Scientific Director), Dr. Petar Tsankov (CEO), Dr. Pavol Belik (Technical Director) and Prof. Martin Vechav (Scientific Director). Image Credits: LatticeFlow
“If you only to look in in data – and this there is a fundamental differentiator for LatticFlow because we are not only to find standard data questions I like it marking questions or poor-quality samples, but too model blind stains, which there is in scripts where in models there is it doesn’t work,” Tsankov explained. “Once in model there is ready we it is possible to take it is eprom different data model questions and to help companies to fix this”.
For example, he noted that models often find hidden correlations that can confound the model and skew the results. In work with an insurance client, for example, who used an ML model to automatically detect dents, scratches, and other damage in images of cars, the model often flagged an image with a finger as a scratch. why? Because in the training set, customers often took a close-up picture of the scratch and pointed to it with their finger. Not surprisingly, the model correlated “finger” with “scratch” even though the car did not have a scratch. The LatticeFlow team argues that these are challenges that go beyond making better labels and need a service that can look at both the model and the training data.
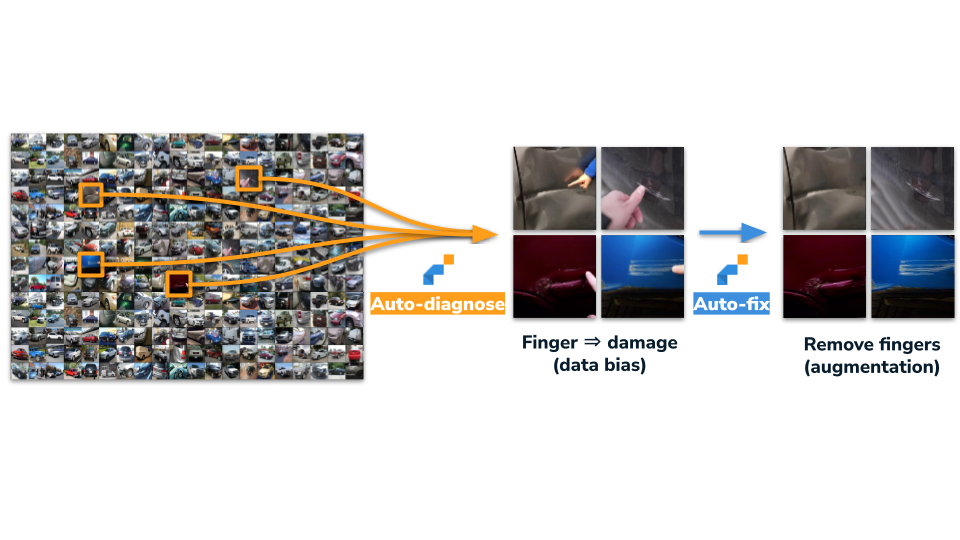
LatticeFlow detects bias in data to train AI models for vehicle damage inspection. Since people often point to scratches, this causes the models to learn that the fingers are pointing to damage (a false indication). This problem is fixed with a custom zoom that removes fingers from all images. Image Credits: LatticeFlow
LatticeFlow itself, it should be noted, does not do training. The service works with pre-prepared models. For now, it’s also focused on offering its services as an on-premise tool, although it may offer a fully managed service in the future as it uses the new funding to aggressively hire both to better serve existing customers and to build its product portfolio.
“The painful truth is that most large-scale AI models today simply do not function reliably in the real world,” said Sunir Kapur, Atlantic Bridge’s operating partner. “Much of this is due to a lack of tools to help engineers effectively address critical AI data and model errors. But this is also why the Atlantic Bridge team made the decision to invest in LatticeFlow so clearly. We believe the company is poised for tremendous growth as it is currently the only company that automatically diagnoses and corrects AI data and model defects at scale.”